Introduction:
In recent years, Generative Artificial Intelligence (AI) has emerged as a captivating field, captivating the imagination of researchers, artists, and technologists alike. With its ability to create novel content across various domains, from realistic images to compelling text, generative AI stands at the forefront of innovation. In this article, we delve into the intricacies of generative AI, exploring its underlying techniques, applications, and the profound impact it has on our world.
Understanding Generative AI:
Generative AI refers to a subset of artificial intelligence techniques designed to generate new content autonomously. Unlike traditional AI systems, which are primarily focused on classification or prediction tasks, generative AI goes beyond mere analysis to create something entirely new. Whether it’s generating lifelike images, composing music, or crafting stories, generative AI models are capable of producing content that mimics human creativity.
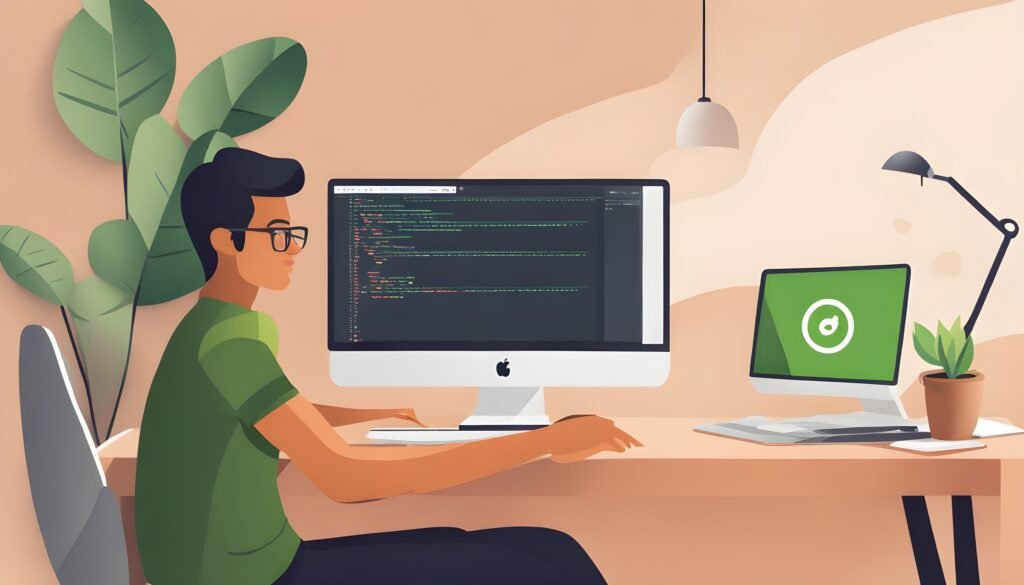
Techniques of Generative AI:
There are several techniques employed in generative AI, each with its unique approach to content generation. Among these, Generative Adversarial Networks (GANs), Variational Autoencoders (VAEs), and Autoregressive Models stand out as prominent methodologies.
- Generative Adversarial Networks (GANs):
- GANs consist of two neural networks: a generator and a discriminator.
- The generator generates synthetic data samples, while the discriminator evaluates the authenticity of these samples.
- Through adversarial training, GANs learn to produce increasingly realistic outputs, indistinguishable from real data.
- Example: StyleGAN, which generates photorealistic images of human faces and landscapes.
- Variational Autoencoders (VAEs):
- VAEs are probabilistic generative models that encode input data into a lower-dimensional latent space.
- They learn to reconstruct the original data from the latent space while maximizing the likelihood of generating the input data.
- VAEs are proficient in tasks such as image generation and reconstruction.
- Example: VAEs can generate diverse variations of images and reconstruct them with fidelity.
- Autoregressive Models:
- Autoregressive models generate data sequentially, conditioning each element on previous elements.
- They are often used in natural language processing tasks for text generation.
- Example: GPT (Generative Pre-trained Transformer) models generate coherent text paragraphs given a prompt.
Applications of Generative AI:
Generative AI finds applications across a diverse array of fields, revolutionizing industries and opening new avenues for creativity and innovation.
- Creative Arts:
- Artists leverage generative AI to explore new artistic styles and push the boundaries of creativity.
- Generative AI aids in generating visual artworks, music compositions, and even poetry.
- Content Creation:
- In media and entertainment, generative AI is used to produce content such as animations, special effects, and virtual environments.
- It enables the rapid creation of synthetic data for training machine learning models, augmenting datasets, and enhancing model performance.
- Healthcare and Drug Discovery:
- Generative AI assists in drug discovery by generating novel molecular structures with desired properties.
- It aids in medical image synthesis, facilitating research and diagnosis in healthcare.
FAQs about Generative AI:
Q: Can generative AI models be controlled?
A: Yes, generative AI models can be controlled by adjusting various parameters during training, such as the learning rate, network architecture, and input data distribution.
Q: Are there ethical concerns associated with generative AI?
A: Yes, ethical considerations such as the misuse of generative AI for creating fake content, deepfakes, and privacy violations are significant concerns that need to be addressed.
Q: How can one get started with generative AI?
A: Beginners can start by exploring introductory tutorials and courses on machine learning and deep learning. Libraries such as TensorFlow and PyTorch provide resources and examples for implementing generative AI models.
Conclusion:
Generative AI holds immense potential to transform industries, spur innovation, and redefine human creativity. As researchers continue to push the boundaries of what’s possible, generative AI will undoubtedly play a pivotal role in shaping the future of technology and human expression. Embracing the power of generative AI opens doors to a world where imagination knows no bounds.